Artificial Intelligence (AI) is revolutionizing the healthcare industry by offering remarkable improvements in efficiency, patient outcomes, and access to care. This transformation is facilitated through various AI types, applications, implications for the workforce, and future prospects. Here, we explore these facets to understand how AI is reshaping healthcare.
Types of Artificial Intelligence in Healthcare
In the realm of healthcare, artificial intelligence (AI) encompasses a broad array of technologies, each designed for specific tasks but collectively aimed at enhancing diagnostics, treatment options, patient care, and research. These technologies include:
Machine Learning (ML): This includes statistical methods such as neural networks and deep learning, enabling algorithms to learn from and make predictions or identify patterns based on data. This is especially critical in precision medicine, allowing for the prediction of treatment plans tailored to individual patient characteristics and situations. From disease classification to detecting cancerous lesions in radiology images, both traditional machine learning and its advanced forms are pivotal in healthcare applications.
Natural Language Processing (NLP): NLP allows computers to comprehend and interpret human language, playing a crucial role in generating, understanding, and classifying clinical documents and research publications. It enhances patient communication, processes clinical notes, and underpins conversational AI technologies, employing both statistical and semantic methods to refine speech and text recognition accuracy.
Robotics: In surgeries and patient care, robotics offers precision and automation. Surgical robots, for instance, augment surgeons’ abilities, facilitating more precise, less invasive operations. Beyond surgical applications, robots are becoming more collaborative and intelligent, capable of working alongside humans and improving through AI advancements.
Computer Vision: This technology enables machines to interpret and analyze images, essential for radiology and pathology. It aids in identifying clinically significant features in imaging data that might be missed by the human eye. Deep learning, a branch of machine learning, significantly enhances diagnostic accuracy in the analysis of oncology images.
Expert Systems and Robotic Process Automation (RPA): These technologies form the foundation of AI’s application in healthcare. Expert systems, which operate on “if-then” rules, provided early AI-based clinical decision support, setting the stage for the more data-driven machine learning algorithms that followed. RPA automates repetitive administrative tasks, simulating human actions in digital processes, and is increasingly integrated with other AI technologies to boost efficiency.
Rather than functioning in isolation, these AI technologies are becoming more interlinked, forming comprehensive solutions in healthcare. Robots, for instance, are being fitted with AI-driven “brains,” and image recognition technologies are being combined with RPA to enhance both service delivery and patient care. The future of AI in healthcare is geared towards a seamless fusion of these technologies, aiming for more inclusive and efficient solutions to healthcare challenges.
Application and Treatment with AI
AI’s involvement in healthcare has seen a remarkable transformation, evolving from the rule-based diagnostic and treatment systems of the 1970s to the cutting-edge applications we see today. These applications span diagnostic assistance, personalized medicine, drug discovery, and remote patient monitoring, marking significant strides in the field:
Diagnostic Assistance: AI algorithms are now pivotal in analyzing medical images, genetic data, and clinical information, enabling early and precise disease detection.
Personalized Medicine: Treatments are increasingly customized, taking into account individual genetic makeup, lifestyle, and environmental influences.
Drug Development: AI accelerates the discovery of new pharmaceuticals by forecasting molecular interactions and potential treatment effects.
Remote Patient Monitoring: The use of wearable technology and sensors for continuous health monitoring represents a leap towards preemptive healthcare.
However, integrating AI into clinical practice poses substantial challenges. Initial excitement around AI systems like IBM Watson, which combines machine learning and natural language processing for cancer care, has diminished. These systems have struggled with specific cancer types and seamless integration into healthcare workflows. Additionally, maintaining rule-based systems within Electronic Health Records (EHR) is complex due to the dynamic nature of medical knowledge and the data surge from genomics and related fields.
The scene is gradually shifting as research institutions and tech companies pioneer the application of AI for diagnostic and therapeutic accuracy. Innovations in radiology, retinal scans, and genomic medicine are underway. Yet, ethical issues and the necessity for integration with clinical operations and EHR systems pose ongoing obstacles.
Tech enterprises and startups are at the forefront, crafting AI algorithms for image analysis, predictive models for high-risk conditions, and cancer treatment plans based on genetic markers. This shift towards evidence- and probability-based medicine underscores the importance of integrating AI into healthcare practices to truly revolutionize diagnosis and treatment. Despite these advancements, the widespread adoption of AI in healthcare is still hampered by integration challenges, highlighting the urgent need for AI technologies that can seamlessly merge with existing healthcare frameworks.
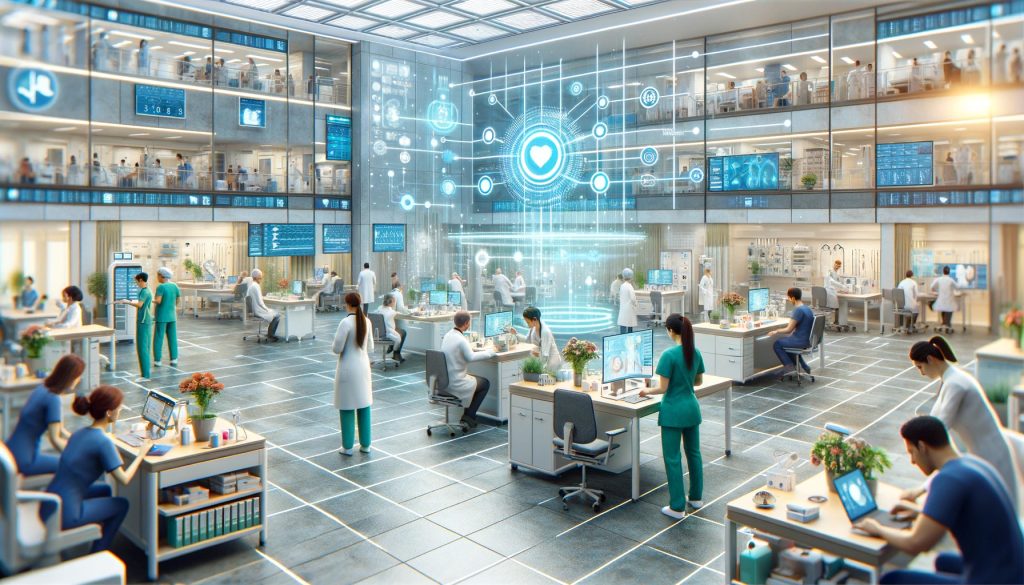
AI Assistance in Diagnosis and Treatment
Artificial Intelligence (AI) is revolutionizing healthcare by enhancing diagnostic precision, optimizing treatment, and fostering personalized medical approaches. This technological evolution addresses long-standing healthcare challenges through:
Early Detection and Diagnosis: AI excels in identifying diseases such as cancer, cardiovascular disorders, and more, with a precision and timeliness surpassing conventional methods. Technologies like Machine Learning (ML) and Deep Learning, especially through Convolutional Neural Networks (CNN), sift through extensive data to pinpoint disease indicators, significantly refining diagnostic accuracy. Research underscores AI’s prowess in lowering both false positives and negatives in mammography, outperforming radiologists in breast cancer detection, identifying skin cancer with remarkable accuracy, and recognizing diabetic retinopathy and cardiovascular diseases with unparalleled precision.
Treatment Optimization and Personalized Medicine: AI enhances patient care by customizing treatment plans through the analysis of data from thousands of patients, factoring in individual genetic makeups, lifestyles, and environmental exposures. This method not only betters patient outcomes but also advances precision medicine by accurately forecasting responses to treatments like chemotherapy and antidepressants. AI’s predictive strength lies in its capacity to analyze intricate data sets, including gene-expression profiles and electronic health records (EHR), aiding clinicians in selecting the most effective therapeutic approaches.
Clinical Laboratory Testing and Microbiology: AI is transforming clinical laboratory testing by elevating the accuracy, speed, and efficiency of identifying microorganisms, diagnosing diseases, and determining antibiotic susceptibilities. The adoption of automation and AI in blood culture analysis, susceptibility testing, and molecular diagnostics has significantly boosted laboratory productivity, facilitating the swift determination of appropriate antibiotic therapies.
Emergency Department (ED) Applications: In the ED, AI assists in triaging patients, highlighting those at high risk, and enhancing patient flow. AI-driven decision support systems provide real-time diagnostic and treatment recommendations, improving resource distribution and treatment choices. This minimizes diagnostic errors, a significant concern in healthcare that can escalate mortality rates and extend hospital stays.
In essence, AI’s role in healthcare is comprehensive, spearheading progress in diagnostics, treatment personalization, laboratory efficiency, and emergency services. Through the application of ML and deep learning, AI is setting the stage for earlier disease detection, tailored healthcare, and heightened efficiency in clinical operations. The continuous evolution and integration of AI into healthcare practices hold the promise of superior patient outcomes, streamlined processes, and empowered healthcare professionals in delivering top-tier care.
Implications for the Workforce
Implications for the healthcare workforce
There has been considerable attention to the concern that AI will lead to automation of jobs and substantial displacement of the workforce. A Deloitte collaboration with the Oxford Martin Institute26 suggested that 35% of UK jobs could be automated out of existence by AI over the next 10 to 20 years. Other studies have suggested that while some automation of jobs is possible, a variety of external factors other than technology could limit job loss, including the cost of automation technologies, labour market growth and cost, benefits of automation beyond simple labour substitution, and regulatory and social acceptance. These factors might restrict actual job loss to 5% or less.
To our knowledge thus far there have been no jobs eliminated by AI in health care. The limited incursion of AI into the industry thus far, and the difficulty of integrating AI into clinical workflows and EHR systems, have been somewhat responsible for the lack of job impact. It seems likely that the healthcare jobs most likely to be automated would be those that involve dealing with digital information, radiology and pathology for example, rather than those with direct patient contact.28
But even in jobs like radiologist and pathologist, the penetration of AI into these fields is likely to be slow. Even though, as we have argued, technologies like deep learning are making inroads into the capability to diagnose and categorise images, there are several reasons why radiology jobs, for example, will not disappear soon.29
First, radiologists do more than read and interpret images. Like other AI systems, radiology AI systems perform single tasks. Deep learning models in labs and startups are trained for specific image recognition tasks (such as nodule detection on chest computed tomography or hemorrhage on brain magnetic resonance imaging). However, thousands of such narrow detection tasks are necessary to fully identify all potential findings in medical images, and only a few of these can be done by AI today. Radiologists also consult with other physicians on diagnosis and treatment, treat diseases (for example providing local ablative therapies) and perform image-guided medical interventions such as cancer biopsies and vascular stents (interventional radiology), define the technical parameters of imaging examinations to be performed (tailored to the patient’s condition), relate findings from images to other medical records and test results, discuss procedures and results with patients, and many other activities.
Second, clinical processes for employing AI-based image work are a long way from being ready for daily use. Different imaging technology vendors and deep learning algorithms have different foci: the probability of a lesion, the probability of cancer, a nodule’s feature or its location. These distinct foci would make it very difficult to embed deep learning systems into current clinical practice.
Third, deep learning algorithms for image recognition require ‘labelled data’ – millions of images from patients who have received a definitive diagnosis of cancer, a broken bone or other pathology. However, there is no aggregated repository of radiology images, labelled or otherwise.
Finally, substantial changes will be required in medical regulation and health insurance for automated image analysis to take off.
Similar factors are present for pathology and other digitally-oriented aspects of medicine. Because of them, we are unlikely to see substantial change in healthcare employment due to AI over the next 20 years or so. There is also the possibility that new jobs will be created to work with and to develop AI technologies. But static or increasing human employment also mean, of course, that AI technologies are not likely to substantially reduce the costs of medical diagnosis and treatment over that timeframe.
Challenges of Working with AI in Healthcare
The integration of Artificial Intelligence (AI) in healthcare brings transformative benefits like improved diagnostics and predictive analytics. However, realizing its full potential involves overcoming significant challenges:
- Data Privacy and Security: Ensuring the confidentiality and integrity of vast personal health information processed by AI systems is crucial. Effective measures are needed to safeguard this data against breaches and misuse while complying with strict regulations.
- Bias and Inequality: Addressing biases in AI algorithms is vital to prevent disparities in treatment outcomes among different demographics. Designing and training AI systems to be unbiased is essential for fair healthcare.
- Regulatory Hurdles: The fast-paced advancement of AI technologies challenges regulatory bodies to develop frameworks that ensure patient safety without hindering innovation. Striking a balance between regulation and technological growth is key.
- Patient Safety and Accuracy: The reliability of AI in making diagnoses and treatment recommendations is critical. AI systems require extensive training to accurately interpret medical data and cater to individual patient needs.
- Integration with Existing IT Systems: Integrating AI technologies into current healthcare IT infrastructures demands a comprehensive understanding of these systems to enhance healthcare workflows effectively.
- Physician Acceptance and Trust: Building trust and acceptance among healthcare providers is essential for the successful implementation of AI. Transparency in AI’s decision-making processes, grounded in current medical research, is necessary to earn physicians’ confidence.
- Compliance with Federal Regulations: Adhering to federal regulations ensures that AI applications in healthcare are ethical, safe, and privacy-compliant.
Addressing these challenges calls for a collaborative effort among tech experts, healthcare professionals, ethicists, and policymakers. Through a focused approach to these issues, the healthcare industry can leverage AI to advance patient care, improve operational efficiency, and foster treatment innovations.
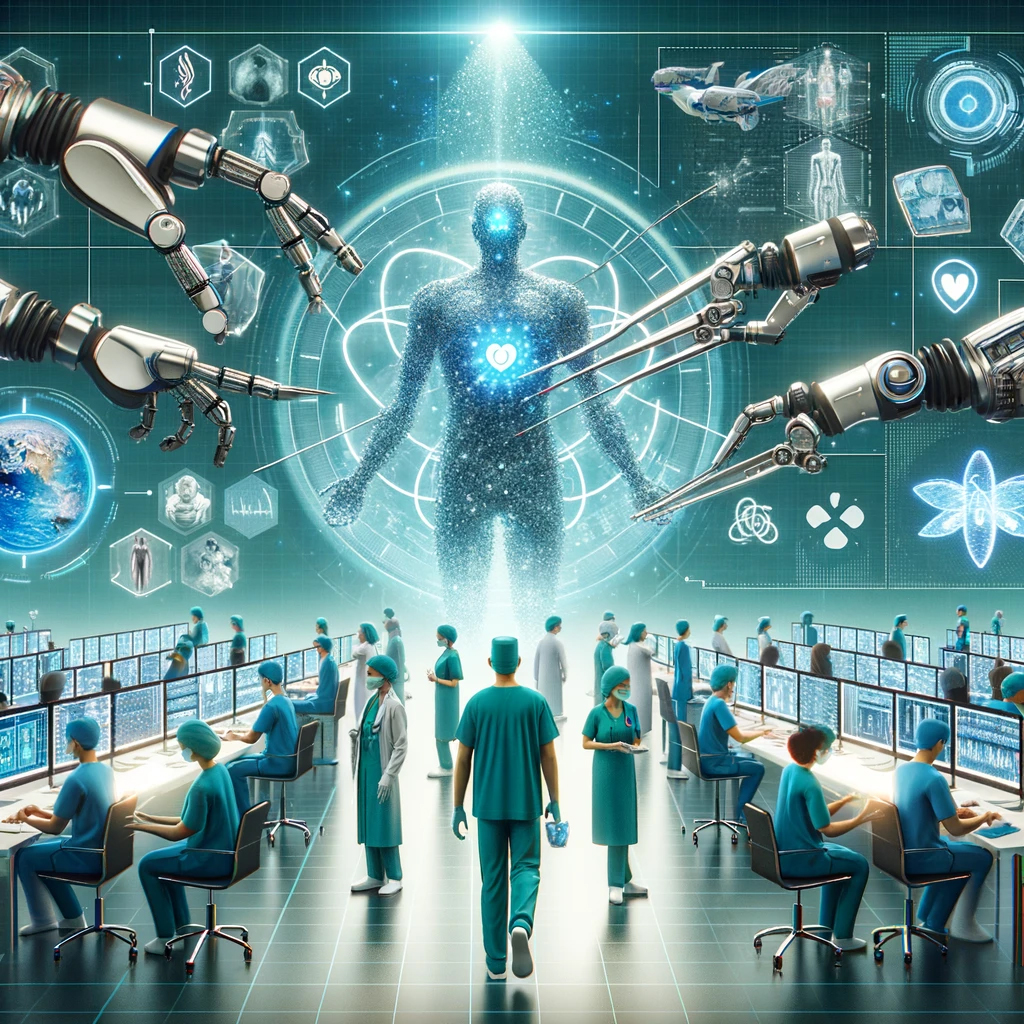
The Future of AI in Healthcare
As artificial intelligence in healthcare has grown in its capabilities, using it to improve medical practices has become increasingly viable. With the development of AI-powered medical tools and intelligent algorithms that are able to interpret large data sets, the potential for using AI in healthcare is limitless. Deep learning AI can be used to help detect diseases faster, provide personalized treatment plans and even automate certain processes such as drug discovery or diagnostics. It also holds promise for improving patient outcomes, increasing safety and reducing costs associated with healthcare delivery.
The future of using artificial intelligence in healthcare is undoubtedly bright and filled with possibilities for further innovation. As we move forward into a more connected digital world, using AI in the healthcare industry will become an invaluable asset that could potentially reshape how doctors treat patients and deliver care. With such great potential, it is clear that using artificial intelligence in healthcare holds the promise of a future filled with advancements, improved health outcomes and better patient experiences.
The greatest challenge to AI in healthcare is not whether the technologies will be capable enough to be useful, but rather ensuring its adoption in daily clinical practice. In time, medical professionals may migrate toward tasks that require unique human skills, tasks that require the highest level of cognitive function. Perhaps the only healthcare providers who will lose out on the full potential of AI in healthcare may be those who refuse to work alongside it.
Benefits of Using AI in Healthcare
Artificial Intelligence (AI) is revolutionizing healthcare, offering significant improvements in how care is delivered and managed. The integration of AI into healthcare systems presents numerous benefits:
- Efficiency and Accuracy: AI boosts healthcare efficiency and accuracy by automating routine tasks and rapidly analyzing vast data volumes. This automation extends to administrative duties, freeing up healthcare professionals to concentrate on patient care.
- Improved Patient Outcomes : AI aids in the early detection of diseases and tailors treatment plans to individual needs, enhancing patient care. Virtual nursing assistants provide constant support, improving patient monitoring and care continuity.
- Cost Reduction : By streamlining healthcare processes and improving service precision, AI significantly reduces operational costs and minimizes the occurrence of unnecessary treatments.
The AI healthcare market’s expected growth to $187 billion by 2030 highlights AI’s increasing importance. Innovations like AI for reducing medication errors and surgical robots demonstrate AI’s potential to enhance safety and outcomes. Furthermore, AI’s application in fraud detection showcases its ability to protect healthcare resources efficiently.
Advancements in machine learning, greater data availability, cost-effective hardware, and the introduction of 5G technology are propelling AI forward in healthcare. These advancements facilitate the rapid analysis of health data, enabling more informed and efficient healthcare delivery.
In essence, AI’s role in healthcare is transformative, promising to enhance operational efficiency, patient outcomes, and cost-effectiveness. As AI technologies evolve and become more integrated into healthcare practices, they promise to redefine the industry, making healthcare more accessible, tailored, and efficient for patients globally.
In conclusion, AI is set to continue its transformative impact on healthcare, offering enhanced care, efficiency, and new opportunities for treatment and diagnosis. However, navigating its challenges requires careful consideration of ethical, regulatory, and workforce implications to fully realize its potential.