In the contemporary digital era, data science stands as a cornerstone in the realms of education and research, wielding a transformative influence that permeates every aspect of these fields. Gone are the days when traditional teaching methods and research practices solely dictated the acquisition and dissemination of knowledge. Instead, data science has ushered in a new era, characterized by innovation, efficiency, and unprecedented insights.
In education, data science has revolutionized teaching methods by providing educators with invaluable tools and methodologies to enhance learning outcomes. Through the analysis of vast datasets encompassing student performance metrics, engagement levels, and learning preferences, educators can gain deep insights into the individual needs and progress of each student. Armed with this information, they can tailor instruction to suit diverse learning styles, thereby fostering a more personalized and effective educational experience.
Moreover, data-driven learning platforms and adaptive technologies leverage sophisticated algorithms to deliver customized learning experiences. By continuously analyzing student interactions and performance data in real-time, these platforms can adapt and refine their content and recommendations to meet the evolving needs of learners. This personalized approach not only enhances engagement but also maximizes learning outcomes, ultimately empowering students to reach their full potential.
In the realm of research, data science has become indispensable in driving scientific discovery and innovation across various disciplines. By harnessing the power of advanced analytics and machine learning algorithms, researchers can analyze complex datasets with unprecedented speed and precision, uncovering hidden patterns, correlations, and insights that pave the way for groundbreaking discoveries.
From genomics and climate science to astronomy and beyond, data science enables researchers to tackle some of the most pressing challenges facing humanity. Whether it’s predicting disease outbreaks, understanding climate change, or exploring the mysteries of the universe, data-driven research methodologies are instrumental in advancing human knowledge and pushing the boundaries of what is possible.
Furthermore, data science facilitates collaboration and knowledge sharing among researchers, breaking down geographic and disciplinary barriers. Cloud-based data repositories and collaboration platforms provide researchers with seamless access to vast repositories of data, enabling them to collaborate on projects and share resources regardless of their location or area of expertise.
Enhancing Learning Outcomes and Personalized Education
Data science is revolutionizing education by providing insights into student performance, preferences, and learning styles. By analyzing vast amounts of educational data, such as assessment scores, attendance records, and engagement metrics, educators can gain valuable insights into student progress and tailor instruction to meet individual needs.
Moreover, data-driven learning platforms and adaptive technologies leverage machine learning algorithms to deliver personalized learning experiences. These platforms analyze student interactions and performance data in real-time to provide customized content, recommendations, and feedback, ultimately enhancing learning outcomes and engagement.
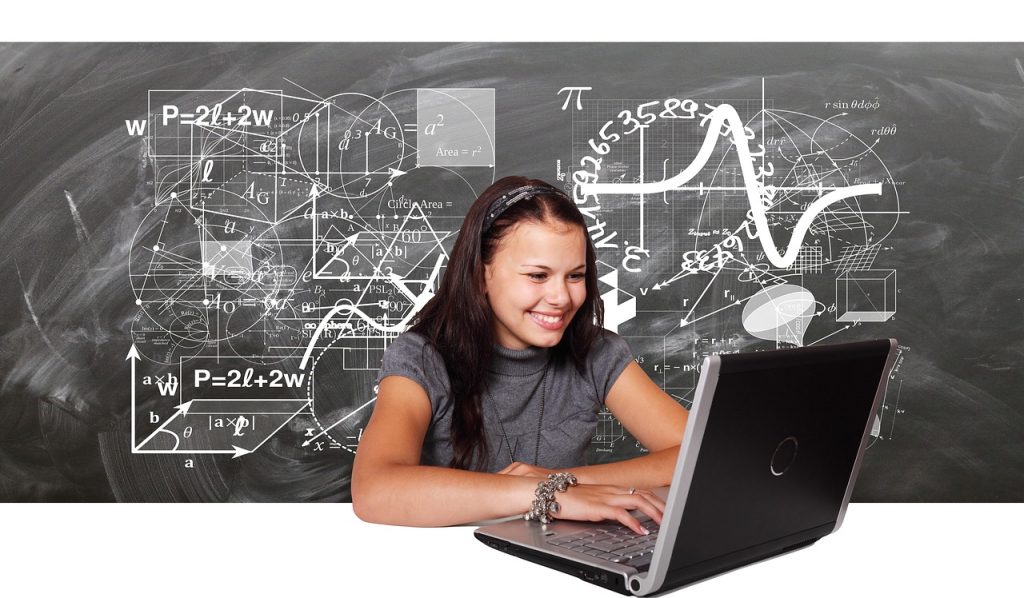
Optimizing Institutional Operations and Resource Allocation
Data science enables educational institutions to optimize their operations and resource allocation. By analyzing administrative data, such as enrollment trends, budget allocations, and facility usage, institutions can identify inefficiencies, streamline processes, and allocate resources more effectively.
Furthermore, predictive analytics models can forecast future enrollment trends, budgetary needs, and staffing requirements, allowing institutions to proactively plan and allocate resources to meet evolving demands. This data-driven approach enhances institutional efficiency, financial sustainability, and overall effectiveness.
Driving Scientific Discovery and Innovation
In the dynamic landscape of scientific research, data science emerges as a pivotal force, propelling groundbreaking discoveries and fostering innovation across various disciplines. By leveraging advanced analytics and machine learning algorithms, researchers can harness the power of data to unlock new insights, uncover hidden patterns, and push the boundaries of human knowledge.
Uncovering Hidden Patterns and Correlations
Data science enables researchers to sift through vast datasets with unprecedented speed and precision, uncovering hidden patterns and correlations that may have otherwise gone unnoticed. Whether analyzing genomic sequences, climate data, or astronomical observations, advanced analytics techniques allow scientists to extract valuable insights that pave the way for groundbreaking discoveries.
Predictive Modeling and Simulation
Predictive modeling, a key component of data science, empowers researchers to forecast future outcomes and simulate complex systems with remarkable accuracy. By developing predictive models based on historical data and scientific principles, researchers can anticipate phenomena ranging from disease outbreaks and natural disasters to the behavior of celestial bodies, enabling proactive measures and informed decision-making.
Collaborative Research and Knowledge Sharing
In an era of global connectivity, data science facilitates collaboration and knowledge sharing among researchers, transcending geographical and disciplinary boundaries. Cloud-based data repositories and collaboration platforms provide scientists with seamless access to vast datasets and computational resources, fostering interdisciplinary collaborations and accelerating the pace of scientific discovery.
Accelerating Drug Discovery and Development
In the field of pharmaceuticals and biotechnology, data science plays a crucial role in accelerating drug discovery and development processes. By analyzing molecular structures, biological pathways, and clinical trial data, researchers can identify promising drug candidates, predict their efficacy and safety profiles, and streamline the drug development pipeline, ultimately bringing life-saving treatments to market more efficiently.
Advancing Materials Science and Engineering
Data science is revolutionizing materials science and engineering by enabling researchers to design novel materials with tailored properties and functionalities. Through computational modeling, data-driven optimization algorithms, and high-throughput experimentation, scientists can expedite the discovery and characterization of new materials for applications ranging from renewable energy and electronics to healthcare and aerospace.
Addressing Societal Challenges and Global Issues
From combating climate change and addressing public health crises to fostering sustainable development and ensuring food security, data science holds immense potential in addressing some of the most pressing challenges facing humanity. By analyzing large-scale environmental data, epidemiological trends, and socioeconomic indicators, researchers can develop data-driven solutions and inform evidence-based policies that promote social welfare and environmental sustainability.
In conclusion, data science serves as a driving force behind scientific discovery and innovation, empowering researchers to unlock new insights, accelerate progress, and tackle complex challenges with unprecedented precision and efficiency. As we continue to harness the power of data science, the possibilities for transformative discoveries and advancements in science and technology are virtually limitless, shaping a brighter and more prosperous future for humanity.
Facilitating Collaborative Research and Data Sharing
Data science facilitates collaborative research and data sharing by providing platforms and tools for researchers to collaborate, share data, and reproduce results. Cloud-based data repositories and collaboration platforms enable researchers to access and share datasets, code, and analyses across geographic and disciplinary boundaries, fostering interdisciplinary collaboration and accelerating scientific progress.
Moreover, data science techniques such as data harmonization and interoperability enable researchers to integrate and analyze heterogeneous datasets from multiple sources. This enables researchers to gain new insights and generate new knowledge by combining data from diverse domains and disciplines.
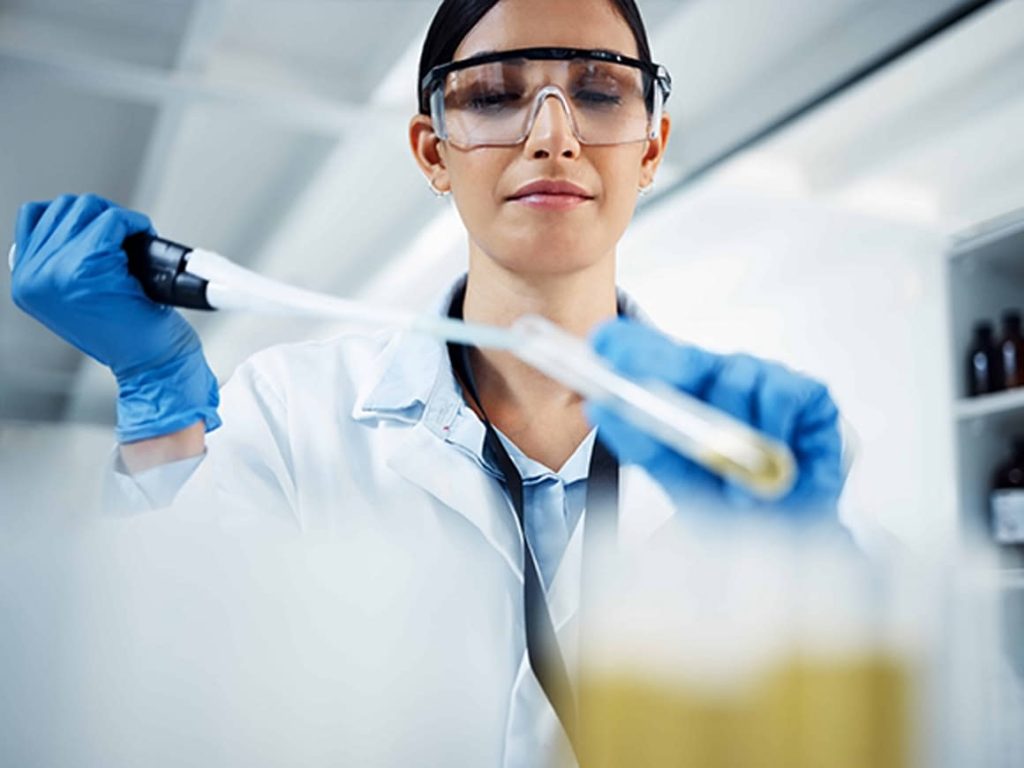
Addressing Ethical and Privacy Considerations
In the realm of data science, ethical and privacy considerations are paramount, particularly in education and research where the handling of sensitive information and personal data is commonplace. As data science continues to advance, it is essential for educators, researchers, and institutions to address these concerns proactively to ensure the responsible and ethical use of data.
Ethical Use of Student Data
In educational settings, data science is often used to analyze student performance, tailor instruction, and improve learning outcomes. However, the collection and analysis of student data raise ethical concerns regarding privacy, consent, and data security. Educators and institutions must establish clear guidelines and protocols for the ethical use of student data, ensuring that data collection is transparent, consent is obtained when necessary, and data is securely stored and anonymized to protect student privacy.
Safeguarding Research Participants
In research settings, data science is instrumental in analyzing large-scale datasets and conducting studies across various domains. However, researchers must prioritize the protection of research participants’ privacy and confidentiality. This involves obtaining informed consent, anonymizing data to prevent the identification of individuals, and implementing robust data security measures to safeguard sensitive information. Additionally, researchers must adhere to ethical guidelines and institutional review board (IRB) regulations to ensure that research activities are conducted ethically and with integrity.
Mitigating Bias and Discrimination
One of the critical challenges in data science is the potential for bias and discrimination in algorithmic decision-making. In educational and research contexts, biased algorithms can perpetuate inequalities and reinforce existing disparities, particularly among marginalized and underrepresented groups. Data scientists and researchers must employ fairness-aware techniques to mitigate bias in data collection, algorithm design, and model deployment. This includes conducting bias audits, analyzing algorithmic outcomes across demographic groups, and implementing fairness constraints to ensure equitable outcomes for all individuals.
Promoting Data Transparency and Accountability
Transparency and accountability are essential principles in data science, particularly concerning educational and research data. Educators and researchers should strive to make their data collection methods, analysis techniques, and research findings transparent and accessible to stakeholders. Open data initiatives, data sharing platforms, and reproducible research practices can enhance transparency and facilitate collaboration while holding researchers accountable for the integrity and reliability of their work.
Fostering Data Literacy and Empowerment
In an era of ubiquitous data collection and analysis, fostering data literacy among students, researchers, and the broader community is crucial. Data literacy empowers individuals to understand, interpret, and critically evaluate data, enabling them to make informed decisions and participate meaningfully in discussions surrounding data-driven issues. Educational institutions and research organizations should prioritize data literacy education and training programs to equip students and researchers with the skills and knowledge necessary to navigate the complexities of the data-driven world responsibly.
Engaging Stakeholders in Ethical Dialogues
Finally, addressing ethical and privacy considerations in data science requires ongoing dialogue and collaboration among stakeholders, including educators, researchers, policymakers, and community members. By engaging in ethical discussions, sharing best practices, and soliciting feedback from diverse perspectives, stakeholders can collectively identify ethical challenges, develop solutions, and establish ethical norms and standards that promote the responsible use of data in education and research.
Conclusion
In conclusion, data science has fundamentally transformed the landscape of education and research, ushering in an era of unprecedented innovation and discovery. From personalized learning experiences to predictive analytics in scientific research, data science has revolutionized how knowledge is acquired, analyzed, and applied.
In education, data science empowers educators to tailor instruction to meet the individual needs of students, fostering deeper engagement and improving learning outcomes. Moreover, data-driven insights enable educational institutions to optimize operations and resource allocation, ensuring efficiency and sustainability.
In the realm of research, data science serves as a catalyst for scientific discovery and innovation, enabling researchers to uncover hidden patterns, simulate complex systems, and collaborate across disciplines. By harnessing the power of data, researchers can address some of the most pressing challenges facing humanity, from understanding the complexities of the human genome to mitigating the impacts of climate change.
However, as data science continues to evolve, it is essential to address ethical and privacy considerations to ensure responsible and ethical use of data. By prioritizing transparency, safeguarding privacy, and engaging stakeholders in ethical dialogues, we can harness the full potential of data science to advance knowledge, drive innovation, and benefit society as a whole.
In essence, data science has become an indispensable tool in the pursuit of knowledge and progress, shaping a brighter and more prosperous future for generations to come.