In the face of mounting challenges such as rising global food demand, climate change impacts, and resource limitations, the agricultural sector is undergoing a profound transformation propelled by data science. This innovative approach encompasses various applications aimed at optimizing production processes and fostering sustainable growth within the industry.
One significant area where data science is making a substantial impact is precision farming. By integrating data from sensors, satellites, and other sources, farmers can monitor crop health, soil conditions, and environmental factors in real-time. This granular level of insight enables precise management of inputs such as water, fertilizers, and pesticides, leading to enhanced resource efficiency and improved crop yields.
Moreover, data science facilitates accurate crop forecasting by analyzing historical data, weather patterns, and market trends. This predictive capability allows farmers to anticipate changes in demand and adjust their production accordingly, reducing the risk of overproduction or shortages and optimizing resource allocation throughout the supply chain.
Additionally, data science is revolutionizing supply chain management in agriculture by optimizing logistics, inventory management, and distribution processes. By leveraging data analytics, stakeholders can gain visibility into supply chain operations, identify inefficiencies, and streamline workflows to ensure timely delivery of fresh, high-quality produce to consumers while minimizing waste and environmental impact.
Precision Agriculture:
Precision agriculture represents a cutting-edge approach to farming that harnesses the power of data science to revolutionize traditional agricultural practices. By integrating data from a diverse array of sources including sensors, satellites, drones, and Internet of Things (IoT) devices, farmers gain real-time insights into crucial factors such as crop health, soil moisture levels, and environmental conditions. This wealth of data allows farmers to make highly informed decisions regarding planting, irrigation, fertilization, and pest control.
The utilization of data science in precision agriculture enables farmers to optimize their farming practices in several ways. For instance, by precisely monitoring crop health and soil conditions, farmers can tailor their irrigation and fertilization strategies to the specific needs of each plant, thereby minimizing water and fertilizer usage while maximizing yields. Additionally, by employing data-driven pest monitoring and control techniques, farmers can proactively address pest infestations, reducing the need for chemical pesticides and minimizing environmental impact.
Overall, precision agriculture powered by data science represents a transformative shift in the way farming is conducted, offering the potential to significantly increase productivity while simultaneously promoting sustainability and environmental stewardship. As technology continues to advance and data science capabilities evolve, the adoption of precision agriculture is expected to become increasingly widespread, ushering in a new era of efficiency and innovation in the agricultural sector.
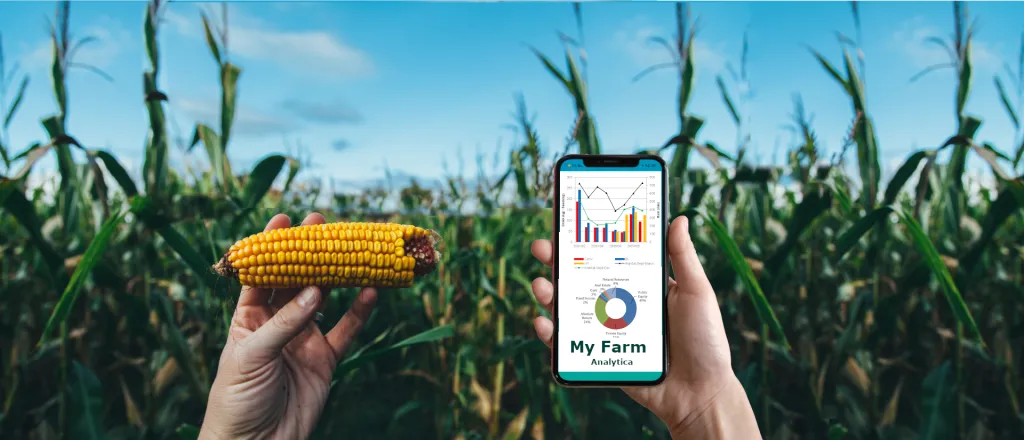
Crop Monitoring and Management:
Crop monitoring and management benefit significantly from the application of data science, empowering farmers to oversee and nurture their crops with unprecedented precision and efficiency. Leveraging satellite imagery, remote sensing technologies, and machine learning algorithms, farmers can detect early indicators of stress, disease, or pest infestation across their fields.
By employing data science techniques, farmers gain real-time insights into crop health and conditions, allowing for timely interventions to mitigate potential threats. These interventions might include adjusting irrigation schedules, applying targeted treatments, or implementing pest control measures precisely where and when they are needed. This proactive approach minimizes crop losses and optimizes yield potential while reducing the reliance on chemical inputs, promoting environmental sustainability in agricultural practices.
Moreover, predictive analytics models play a vital role in crop management by forecasting yields, quality, and market demand. By analyzing historical data and current trends, farmers can anticipate future outcomes and make informed decisions regarding harvest timing and resource allocation. This allows for optimized production planning, ensuring that crops are harvested at their peak quality and delivered to market when demand is highest.
Smart Irrigation:
Water scarcity poses a considerable challenge to agriculture, particularly in the face of climate change and population growth. However, data science provides innovative solutions for smart irrigation management, enabling farmers to optimize water usage while sustaining crop health and productivity. By integrating various technologies such as soil moisture sensors, weather forecasts, and predictive analytics algorithms, farmers can make informed decisions about irrigation practices to minimize water waste and enhance water efficiency on farms.
The utilization of soil moisture sensors allows farmers to accurately assess soil moisture levels in real-time, providing insights into when and where irrigation is needed most. Additionally, weather forecasts help farmers anticipate precipitation events and adjust irrigation schedules accordingly to avoid overwatering or underwatering crops. Furthermore, predictive analytics algorithms analyze historical data on soil moisture, weather patterns, and crop water requirements to forecast optimal irrigation schedules and volumes, ensuring that crops receive the right amount of water at the right time.
Supply Chain Optimization:
Smart Supply chain optimization in agriculture benefits immensely from the application of data science, facilitating the efficient flow of goods from farm to fork. By integrating data from various stakeholders including farmers, suppliers, distributors, retailers, and consumers, supply chain participants gain comprehensive visibility into inventory levels, demand fluctuations, and transportation logistics.
Through data analytics and predictive modeling, stakeholders can anticipate changes in demand and optimize inventory management to meet consumer needs effectively. This proactive approach minimizes the risk of overstocking or shortages, reducing waste and maximizing resource utilization throughout the supply chain.
Furthermore, data science enables stakeholders to optimize transportation logistics by analyzing route optimization, vehicle scheduling, and delivery times. By leveraging data-driven insights, transportation operations can be streamlined to ensure the timely delivery of fresh, high-quality produce to consumers while minimizing transportation costs and environmental impact.
Sustainable Farming Practices:
Data science plays a crucial role in facilitating the adoption of sustainable farming practices by providing valuable insights into environmental impacts and resource usage. Through the analysis of data related to soil health, biodiversity, carbon sequestration, and greenhouse gas emissions, farmers can make informed decisions to implement regenerative agriculture techniques that promote sustainability.
By leveraging data science, farmers can gain a deeper understanding of soil health parameters such as nutrient levels, pH balance, and organic matter content. This enables them to tailor soil management practices to enhance soil fertility, improve water retention, and reduce erosion, thereby promoting long-term agricultural sustainability.
Moreover, data-driven insights into biodiversity and ecosystem services allow farmers to implement practices that support habitat conservation, pollinator populations, and natural pest control. This may include the adoption of agroforestry systems, the creation of wildlife habitats, and the preservation of natural vegetation corridors.
Additionally, data science enables farmers to optimize crop rotations, cover cropping, and integrated pest management strategies to minimize reliance on chemical inputs and promote ecological balance. By analyzing historical data on pest populations, weather patterns, and crop yields, farmers can make data-driven decisions to mitigate pest pressure and optimize resource use.
Conclusion
In conclusion, the agricultural sector is undergoing a profound transformation fueled by the integration of data science, which offers innovative solutions to address mounting challenges such as rising global food demand, climate change impacts, and resource limitations. Through precision agriculture, farmers can optimize production processes by leveraging real-time data on crop health, soil conditions, and environmental factors, leading to enhanced resource efficiency and improved yields. Additionally, data science enables accurate crop forecasting and supply chain optimization, ensuring timely delivery of high-quality produce to consumers while minimizing waste and environmental impact.
Furthermore, data-driven insights empower farmers to adopt sustainable farming practices that promote soil health, biodiversity, and ecological balance. By leveraging data science, farmers can make informed decisions regarding irrigation, fertilization, pest control, and crop rotation, minimizing reliance on chemical inputs and fostering long-term agricultural sustainability.
Overall, the integration of data science into agriculture holds immense potential to revolutionize the industry, driving efficiency, productivity, and sustainability. As technology continues to advance and data science capabilities evolve, the adoption of data-driven approaches is expected to become increasingly widespread, ushering in a new era of innovation and growth in the agricultural sector.