Data science enables predictive maintenance and asset management by analyzing sensor data, equipment performance metrics, and historical maintenance records to anticipate equipment failures and optimize maintenance schedules. Machine learning algorithms can identify patterns and anomalies in sensor data, allowing engineers to predict when components are likely to fail and schedule maintenance proactively. Predictive maintenance minimizes downtime, reduces maintenance costs, and extends asset lifecycles, ensuring optimal performance and reliability of critical infrastructure and equipment.
Design Optimization and Simulation
Data science facilitates design optimization and simulation by leveraging computational modeling, simulation techniques, and optimization algorithms to improve product design and performance. Engineers can use data-driven approaches to explore a wide range of design alternatives, evaluate their performance under different conditions, and identify optimal design configurations. By analyzing simulation results and experimental data, engineers can iteratively refine designs, reduce development time, and enhance product quality and functionality.
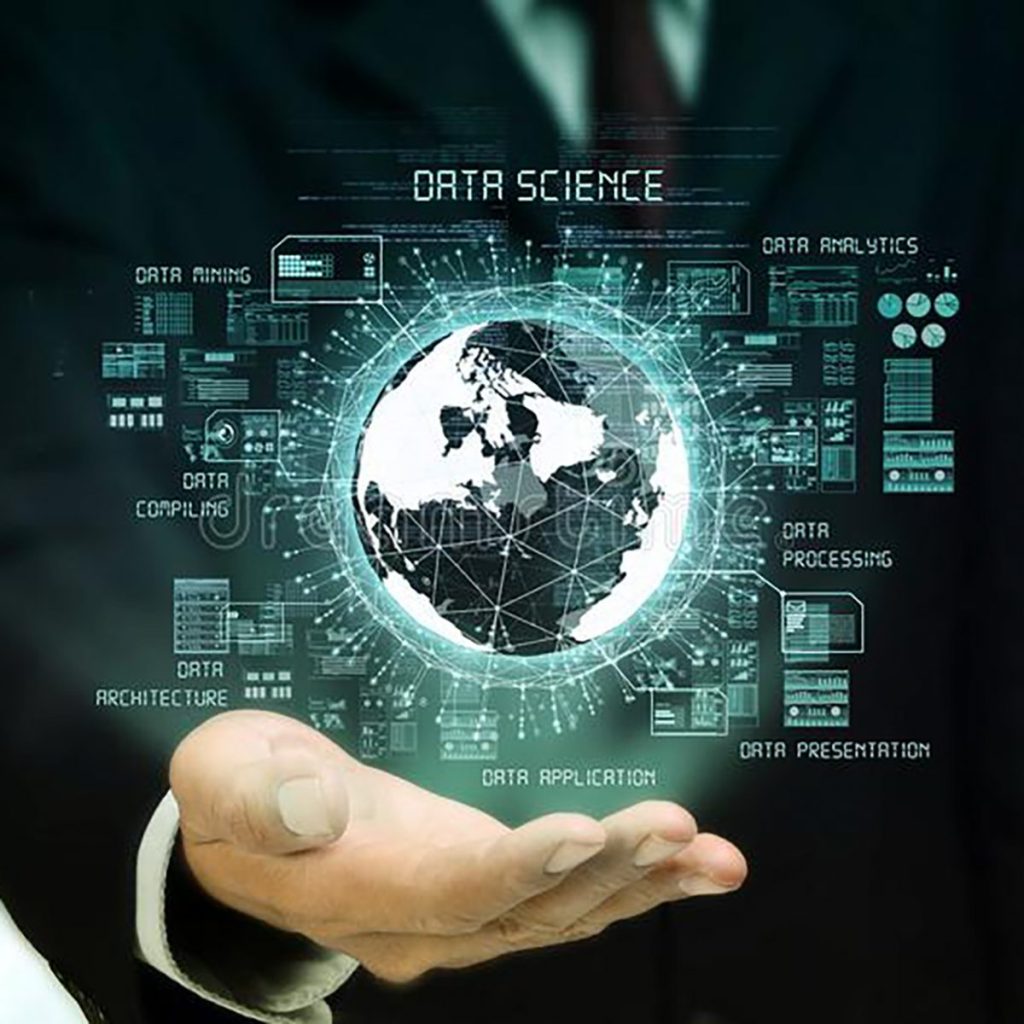
Smart Manufacturing and Process Optimization
Data science drives smart manufacturing and process optimization initiatives by integrating sensor data, real-time monitoring systems, and advanced analytics to improve production efficiency and quality. Machine learning algorithms can analyze production data to identify inefficiencies, detect anomalies, and optimize process parameters in real-time. Smart manufacturing technologies enable adaptive control and predictive maintenance, allowing manufacturers to reduce waste, minimize defects, and increase throughput while maintaining product quality and consistency.
Energy Efficiency and Sustainability
Data science plays a crucial role in promoting energy efficiency and sustainability in engineering applications, such as building management systems, renewable energy systems, and transportation networks. Engineers can use data analytics to monitor energy consumption, identify energy-saving opportunities, and optimize energy usage patterns. Machine learning algorithms can predict energy demand, optimize renewable energy generation, and optimize energy storage systems to maximize efficiency and reduce environmental impact.
Structural Health Monitoring and Safety Analysis
Data science enables structural health monitoring and safety analysis by analyzing sensor data, structural performance metrics, and historical inspection records to assess the condition of infrastructure assets and identify potential safety risks. Machine learning algorithms can detect structural anomalies, predict future failures, and prioritize maintenance and repair activities. Structural health monitoring technologies enhance safety, reliability, and resilience of critical infrastructure, such as bridges, dams, and buildings, ensuring public safety and minimizing the risk of catastrophic failures.
Supply Chain Management and Logistics Optimization
Data science drives supply chain management and logistics optimization efforts by analyzing supply chain data, transportation routes, and inventory levels to improve efficiency and reduce costs. Engineers can use data analytics to optimize inventory levels, minimize stockouts, and streamline transportation and distribution processes. Machine learning algorithms can forecast demand, identify supply chain disruptions, and optimize procurement and production schedules to meet customer-mandated efficiency and minimize delivery times.
Quality Control and Defect Detection
Data science enables quality control and defect detection in manufacturing processes by analyzing production data, sensor readings, and quality inspection results to identify defects and deviations from quality standards. Engineers can use data analytics to monitor product quality in real-time, detect defects early in the production process, and implement corrective actions to minimize defects and rework. Machine learning algorithms can classify defects, predict defect occurrences, and optimize quality control processes to ensure consistent product quality and customer satisfaction.
Process Automation and Robotics
Data science drives process automation and robotics initiatives by integrating sensor data, machine learning algorithms, and robotic systems to automate repetitive tasks and improve operational efficiency. Engineers can use data analytics to optimize robot trajectories, plan robot movements, and coordinate multi-robot systems in complex manufacturing environments. Process automation technologies enable flexible manufacturing, agile production, and adaptive manufacturing processes, allowing engineers to respond quickly to changing customer demands and market conditions.
Conclusion
Data science stands as a transformative force across various engineering disciplines, dramatically enhancing efficiency, quality, and innovation. By harnessing the power of big data, analytics, and machine learning, engineers are equipped to predict failures before they occur, optimize processes in real-time, and design products that are both innovative and reliable. Whether through smarter manufacturing practices, sustainable energy use, or advanced robotics, the applications of data science in engineering not only drive technological advancement but also foster economic growth and environmental sustainability. As industries continue to evolve, the role of data science will likely expand, further integrating into the fabric of engineering to tackle complex challenges and meet the demands of a rapidly changing world.