The integration of Artificial Intelligence (AI) into academic research holds immense promise for transforming various fields, yet it also introduces a host of challenges and ethical dilemmas that necessitate careful consideration. Here, we explore the multifaceted landscape of challenges and ethical considerations in AI-driven academic research, drawing insights from existing literature and augmenting them with additional perspectives.
Bias and Fairness
AI algorithms, reliant on training data, can inadvertently amplify biases inherent in the data, leading to skewed outcomes and exacerbating existing disparities. For example, if the data used to train an AI model predominantly represents a particular demographic, the model’s predictions may disproportionately favor that group, resulting in biased conclusions. This issue is particularly concerning in sensitive domains like healthcare and criminal justice, where biased AI systems can perpetuate inequality and injustice.
To mitigate these biases, researchers must diligently curate and preprocess data to foster fairness and counteract inherent biases. This involves diversifying the datasets, applying bias correction techniques, and continuously monitoring AI outputs for any indications of unfairness. Additionally, adopting a multidisciplinary approach that incorporates insights from ethics, sociology, and data science can help create more equitable AI systems. Collaborative efforts and continuous dialogue among various stakeholders are essential to address and reduce biases effectively.
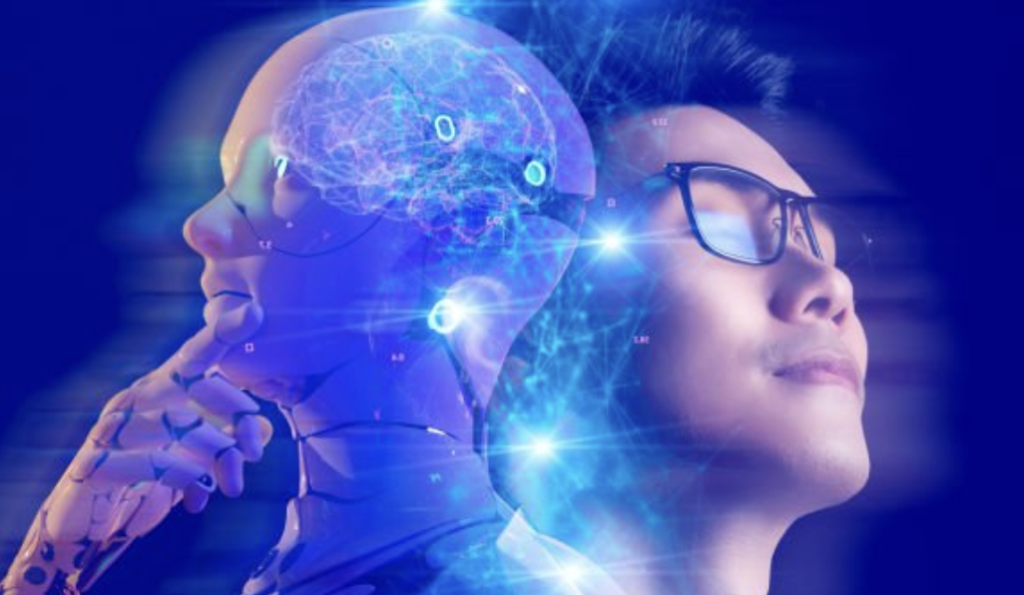
Transparency and Interpretability
The opacity of certain AI algorithms, notably deep learning models, poses challenges to understanding and interpreting their decision-making processes. These “black box” models, while powerful, often operate in ways that are not easily understandable to humans, making it difficult to validate their findings or trust their conclusions. This lack of transparency can hinder the reproducibility of research and erode trust in AI-driven results.
Enhancing transparency in AI-driven research is imperative, necessitating the development of methods to elucidate the rationale behind AI-generated results. Techniques such as explainable AI (XAI) aim to make AI systems more interpretable by providing clear and understandable explanations of how decisions are made. Researchers can leverage these methods to foster trust and reproducibility in academic inquiry, ensuring that AI models are not only accurate but also comprehensible. Increased transparency also facilitates collaboration and knowledge sharing, which are vital for the scientific community.
Privacy and Data Protection
The proliferation of AI-driven research engenders concerns regarding the handling of vast troves of sensitive data. AI systems often require large datasets, which can include personal and sensitive information, raising significant privacy issues. Ensuring robust privacy safeguards and informed consent mechanisms is crucial to protect individuals’ data and maintain ethical standards.
Researchers must navigate intricate regulatory frameworks, such as the General Data Protection Regulation (GDPR) in Europe, to ensure compliance with data protection laws. This involves implementing strong data anonymization techniques, securing data storage, and obtaining explicit consent from data subjects. Upholding these standards not only protects individuals’ privacy but also reinforces the ethical integrity of AI-driven research. Effective data protection practices help maintain public trust and encourage greater participation in research studies.
Accountability and Responsibility
As AI systems assume greater autonomy, questions surrounding accountability and liability come to the fore. When AI-driven interventions lead to unintended consequences or adverse outcomes, determining who is responsible can be complex. Clear delineation of responsibilities among researchers, developers, and other stakeholders is essential to address these challenges.
Researchers bear the onus of preemptively identifying and addressing potential risks associated with their AI systems. This involves conducting thorough risk assessments, engaging in scenario planning, and establishing protocols for mitigating adverse effects. By proactively managing these responsibilities, researchers can safeguard against negative impacts and ensure that AI-driven research adheres to ethical standards. Establishing accountability frameworks promotes transparency and ethical behavior in AI development and deployment.
Ethical Use of AI
Ethical considerations in AI-driven research extend beyond technical domains to encompass broader societal implications. Researchers must grapple with questions of fairness, equity, and justice throughout the AI lifecycle, from design and deployment to evaluation. Ensuring that AI research endeavors serve the collective good while mitigating harm to vulnerable populations is a fundamental ethical imperative.
This involves adopting a holistic approach to AI development that prioritizes the well-being of all stakeholders. Researchers should engage with diverse communities to understand their needs and perspectives, incorporate ethical guidelines into their workflows, and strive for transparency and accountability in their research practices. By doing so, they can create AI systems that are not only effective but also ethically sound. Ethical AI use also requires ongoing reflection and adaptation to evolving societal norms and values.
Dual-Use and Misuse
The dual-use potential of AI technologies introduces ethical quandaries regarding their potential for both constructive and deleterious applications. AI systems designed for beneficial purposes, such as improving healthcare diagnostics, can also be repurposed for harmful uses, such as creating deepfakes or conducting surveillance. Researchers must exercise vigilance to anticipate and mitigate the risks of misuse.
Implementing robust ethical review processes and fostering a culture of responsible innovation are key strategies to address these challenges. By anticipating potential risks and developing safeguards, researchers can minimize the likelihood of AI technologies being misused. This proactive approach helps ensure that AI advances contribute positively to society and do not exacerbate existing problems or create new ethical dilemmas. Vigilance in monitoring AI applications is crucial to prevent their abuse.
Human-Centric Design
Central to AI-driven academic research is the imperative of prioritizing human welfare and dignity. A human-centric approach to AI design and development involves embedding principles of transparency, accountability, fairness, and inclusivity into AI systems. This approach ensures that AI technologies are developed with a focus on human rights and values.
By prioritizing human-centric design, researchers can create AI systems that respect individuals’ autonomy and enhance their quality of life. This involves engaging with stakeholders throughout the development process, addressing ethical concerns, and ensuring that AI systems are accessible and beneficial to all. Ultimately, a human-centric approach to AI fosters trust and supports the responsible deployment of AI technologies in research. Engaging with end-users and other stakeholders early in the design process can lead to more effective and accepted AI solutions.
Ensuring Inclusivity and Diversity
Inclusivity and diversity are critical to the ethical deployment of AI in research. AI systems developed without considering diverse perspectives can reinforce existing biases and exclude marginalized groups. Ensuring that AI research includes diverse voices and addresses the needs of underrepresented communities is essential for creating equitable technologies.
Researchers should strive to build diverse teams and engage with a broad range of stakeholders to understand different viewpoints and needs. This approach helps identify and address potential biases in AI systems and ensures that the benefits of AI are shared widely. By fostering inclusivity and diversity, researchers can contribute to the development of AI technologies that are fair and just. Promoting diversity in research teams also enhances creativity and innovation, leading to more robust AI solutions.
Addressing Environmental Impact
AI-driven research also raises concerns about the environmental impact of deploying large-scale computing resources. Training AI models often requires significant computational power, leading to high energy consumption and carbon emissions. Addressing the environmental footprint of AI research is an emerging ethical consideration that researchers must navigate.
To mitigate the environmental impact, researchers can adopt more energy-efficient algorithms, utilize renewable energy sources, and optimize computational resources. Additionally, raising awareness about the environmental implications of AI and incorporating sustainability into research practices can help reduce the carbon footprint of AI technologies. Balancing the benefits of AI with its environmental impact is crucial for sustainable and ethical AI research. Researchers must also advocate for and support policies that promote sustainable computing practices.
Long-Term Societal Impacts
The long-term societal impacts of AI-driven research are complex and multifaceted. While AI holds the potential to drive significant advancements across various fields, it also poses risks that could affect societal structures and individual lives. Researchers must consider the broader implications of their work and strive to anticipate and address long-term consequences.
Engaging in interdisciplinary collaborations and continuous dialogue with policymakers, ethicists, and the public can help identify and mitigate potential risks. By adopting a forward-looking perspective and considering the societal impacts of AI, researchers can contribute to the development of AI technologies that promote social good and minimize harm. Ensuring that AI advances are aligned with societal values and ethical principles is essential for sustainable and responsible innovation. Long-term planning and scenario analysis can help researchers anticipate and address future challenges.
Conclusion
The integration of AI into academic research brings significant opportunities and challenges. Addressing the ethical considerations and potential risks associated with AI is essential to ensure that its benefits are realized while minimizing adverse impacts. By focusing on bias and fairness, transparency and interpretability, privacy and data protection, accountability and responsibility, and other ethical aspects, researchers can harness the transformative potential of AI in a manner that is ethical, inclusive, and beneficial to society. As AI continues to evolve, ongoing vigilance and commitment to ethical principles will be crucial for guiding its development and application in academic research.