Artificial Intelligence (AI) stands as the pinnacle of human innovation, representing the fusion of computer science, mathematics, and cognitive psychology. At its core, AI seeks to replicate human intelligence in machines, enabling them to perform tasks that traditionally require human cognition. This encompasses a wide range of capabilities, including problem-solving, learning, reasoning, and perception. What sets AI apart is its ability to adapt and improve its performance over time, a characteristic known as machine learning, which forms the foundation of many AI applications today.
One of the fundamental concepts driving AI is neural networks, which are inspired by the structure and function of the human brain. These networks consist of interconnected nodes, or artificial neurons, organized into layers that process information through weighted connections. Through training on vast datasets, neural networks can learn to recognize patterns and make predictions, mimicking the way humans learn from experience. Deep learning, a subset of machine learning, harnesses the power of neural networks with multiple layers, enabling complex tasks such as image and speech recognition with remarkable accuracy.
Beyond its technical intricacies, AI holds immense potential to transform industries and enhance various aspects of human life. From healthcare to finance, AI-powered systems are streamlining processes, optimizing decision-making, and unlocking insights from massive amounts of data. However, alongside its promise comes ethical and societal considerations, including concerns about job displacement, data privacy, and algorithmic bias. As we continue to push the boundaries of AI technology, it’s imperative to foster a deep understanding of its capabilities and implications, ensuring that AI serves as a force for positive change in the world. But what exactly is AI, and how does it work? Let’s delve into this fascinating field and explore its various facets.
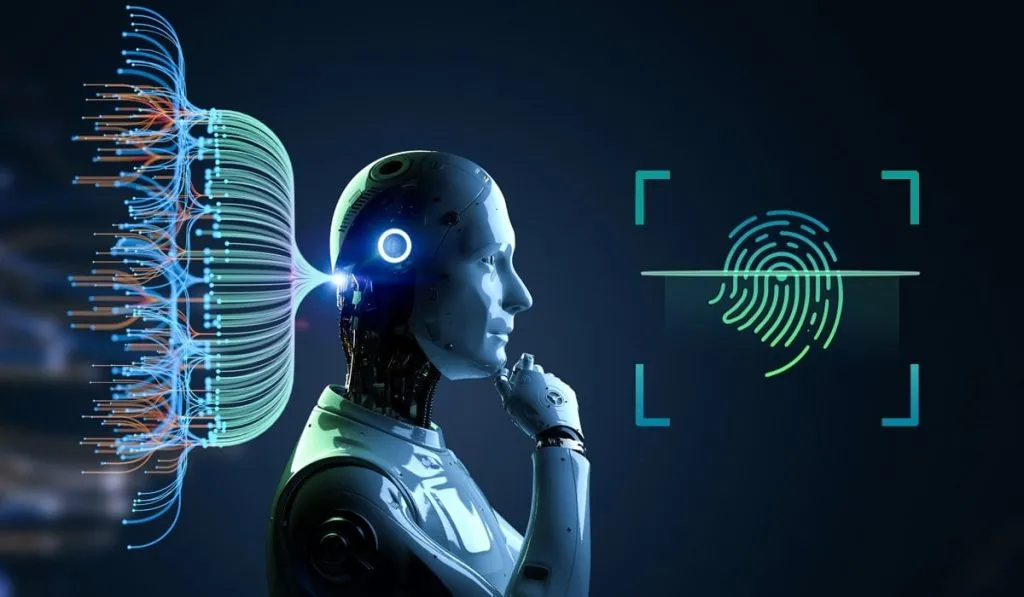
What is Artificial Intelligence?
Artificial Intelligence (AI) stands at the forefront of modern technology, representing the culmination of decades of research and innovation in computer science. At its essence, AI encompasses a diverse array of techniques and approaches aimed at imbuing machines with capabilities traditionally associated with human intelligence. These capabilities include but are not limited to learning from data, reasoning to solve complex problems, recognizing patterns in vast datasets, understanding natural language, and even exhibiting forms of creativity. Through the development of AI systems, humans seek to replicate and enhance their own cognitive abilities in machines, opening up new frontiers of possibility in automation and decision-making.
Central to the concept of AI is the notion of machine learning, a subfield that focuses on creating algorithms and models capable of learning from data and improving their performance over time. Machine learning techniques enable AI systems to detect patterns, make predictions, and generate insights from large volumes of information with unprecedented accuracy and efficiency. Supervised learning, unsupervised learning, and reinforcement learning are among the key paradigms within machine learning, each offering unique approaches to training AI models and solving different types of problems. By harnessing the power of machine learning, AI systems can adapt to changing environments, refine their strategies, and achieve increasingly sophisticated levels of autonomy.
The pervasive impact of AI is felt across numerous industries and sectors, driving transformative changes in how businesses operate, how healthcare is delivered, how transportation systems function, and how societies interact with technology. From virtual assistants and recommendation systems to autonomous vehicles and medical diagnostic tools, AI applications continue to revolutionize the way we live, work, and communicate.
Types of AI
Types of AI can be classified into two main categories: Narrow AI and General AI.
Narrow AI: Also known as Weak AI, Narrow AI is designed to perform specific tasks within a limited domain. Examples of Narrow AI include virtual assistants like Siri and Alexa, recommendation algorithms used by streaming services, and facial recognition software.
General AI: General AI, or Strong AI, refers to a hypothetical system with the ability to understand, learn, and apply knowledge across a wide range of tasks, similar to human intelligence. While General AI remains a long-term goal for researchers, it has yet to be achieved.
How Does AI Work?
Understanding the Mechanisms of AI involves a network of algorithms—sophisticated sets of mathematical instructions—that dictate how AI systems operate. These algorithms serve as the blueprints guiding the behavior of AI, allowing them to process input data, identify patterns, and generate output in response to specific tasks. Whether it’s recognizing faces in images, understanding spoken language, or making recommendations based on user preferences, AI algorithms provide the computational framework for these tasks to be accomplished.
Machine learning stands as a pivotal subset of AI, enabling systems to evolve and adapt through experience. In traditional programming, developers explicitly code instructions for a computer to follow. In contrast, machine learning algorithms learn from data, continually refining their performance without explicit programming. Through the iterative process of training on large datasets, machine learning models adjust their parameters to recognize patterns and make predictions. This capability allows AI systems to improve their accuracy and effectiveness over time, enhancing their ability to tackle complex tasks across various domains.
The concept of learning from data forms the cornerstone of machine learning, with different approaches such as supervised learning, unsupervised learning, and reinforcement learning offering distinct methodologies for training AI models. Supervised learning involves training a model on labeled data, where the correct outputs are provided, allowing the model to learn from the examples and make predictions on new, unseen data.
Unsupervised learning, on the other hand, involves training on unlabeled data, where the model must infer the underlying structure or patterns without explicit guidance. Reinforcement learning operates on a reward-based system, where the AI agent learns to optimize its actions based on feedback from the environment. Together, these machine learning techniques empower AI systems to tackle a wide array of tasks, paving the way for innovative applications across industries and domains.
Applications of AI
Artificial Intelligence has numerous applications across various industries, transforming businesses, healthcare, education, transportation, and more.
- Ai in Business: AI-powered analytics help businesses make data-driven decisions, improve customer service, and automate routine tasks.
- Ai in Healthcare: AI aids in disease diagnosis, drug discovery, personalized medicine, and remote patient monitoring.
- Ai in Education: AI technologies enhance learning experiences through personalized tutoring, adaptive learning platforms, and intelligent educational tools.
- Ai in Transportation: Self-driving cars and predictive maintenance systems are examples of AI applications in transportation, aiming to improve safety and efficiency.
Ethical Considerations
As Artificial Intelligence (AI) continues to permeate various aspects of society, ethical considerations loom large, prompting critical reflections on its implications. Algorithmic bias, for instance, raises concerns about AI systems perpetuating or exacerbating societal inequalities by favoring certain groups or demographics over others. This bias can emerge from the data used to train AI models, reflecting historical prejudices or systemic disparities. Similarly, privacy violations arise as AI systems collect and analyze vast amounts of personal data, raising questions about consent, transparency, and individual autonomy. Safeguarding privacy rights in the age of AI necessitates robust regulations and ethical guidelines to ensure responsible data usage and protection.
Another pressing ethical concern revolves around job displacement, as automation driven by AI technologies threatens to disrupt traditional employment sectors. While AI promises efficiency gains and new opportunities, it also poses challenges for workers whose jobs are rendered obsolete or significantly altered. Addressing these challenges requires proactive measures such as retraining programs, social safety nets, and policies that promote inclusive economic growth. Moreover, the prospect of autonomous weapons systems raises ethical dilemmas regarding the delegation of lethal decision-making to AI algorithms. Striking a balance between military innovation and ethical responsibility entails international cooperation, legal frameworks, and ethical standards to prevent the misuse of AI in warfare and ensure human oversight in critical decision-making processes.
Mitigating these ethical challenges necessitates interdisciplinary collaboration among technologists, policymakers, ethicists, and stakeholders from diverse backgrounds. Establishing responsible AI frameworks involves not only technical considerations but also ethical principles such as fairness, accountability, transparency, and societal impact. By fostering dialogue and engagement across various stakeholders, we can navigate the ethical complexities of AI deployment, promote ethical AI research and development, and cultivate a future where AI serves the collective good while upholding fundamental human values and rights.
The Future of AI
In the realm of healthcare, AI is poised to revolutionize diagnosis, treatment, and patient care. Machine learning algorithms can analyze vast amounts of medical data to identify patterns and predict diseases with unprecedented accuracy. Additionally, AI-powered robotics are being developed to assist surgeons in delicate procedures, enhancing precision and reducing the risk of human error. With AI, personalized medicine tailored to individual genetic makeup and lifestyle factors could become commonplace, leading to more effective treatments and improved patient outcomes.
However, the future of AI also raises important ethical and societal considerations that must be addressed. Concerns about job displacement due to automation, algorithmic bias, privacy infringements, and the concentration of power in the hands of a few tech giants loom large. To fully harness the benefits of AI while mitigating its risks, it is crucial to prioritize ethical AI development, promote transparency and accountability in algorithmic decision-making, and ensure that AI technologies are deployed in ways that uphold human rights and promote the common good. Only by approaching AI with foresight, responsibility, and inclusivity can we shape a future where AI serves as a powerful tool for positive societal transformation.
Conclusion
In conclusion, the future of AI holds both tremendous promise and profound challenges. As we continue to push the boundaries of AI technology, it’s essential to recognize its potential to address complex societal issues, revolutionize industries, and enhance human well-being. From healthcare and education to business and transportation, AI has the power to drive innovation and transformation on a global scale. However, realizing this potential requires us to navigate ethical considerations, such as algorithmic bias, job displacement, and privacy concerns, with diligence and foresight.
In essence, the future of AI is not predetermined but shaped by the choices we make today. By approaching AI with a commitment to responsible innovation and ethical stewardship, we can pave the way for a future where AI serves as a powerful tool for advancing human progress and creating a more equitable and sustainable world.